A stylized use of sparse autoencoders reveals how models, like those recognizing Paris as the City of Light, process information. While interpretability researchers initially hoped for a direct mapping between activations and individual neurons, practical challenges arose due to neurons activating for multiple, unrelated features. Sparse autoencoders address this by isolating a small number of features from complex activations, facilitating the identification of underlying model features without predefined input. This approach uncovers unexpected structures and patterns, providing insights into model behavior through unanticipated features highlighted by activation strength.
Gemma Scope Unveiling the Complexities of Language Models to Support Safety Experts
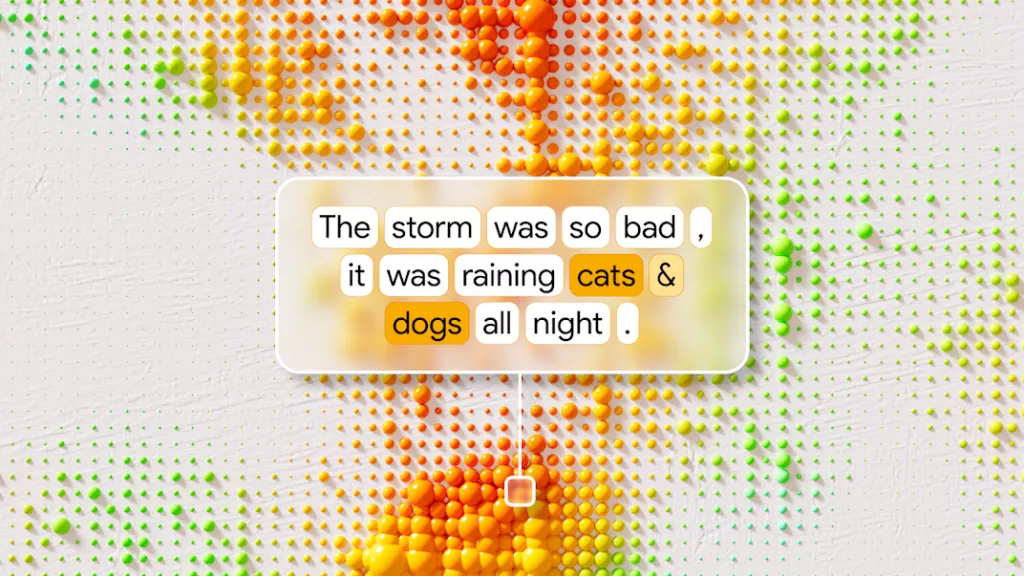